Leveraging AI in the Banking Industry: A guide for CFOs
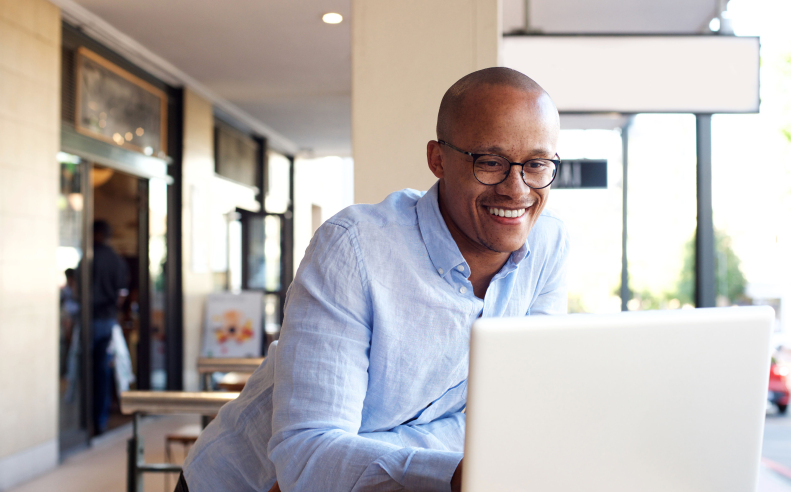
The highly responsive capabilities of generative AI have put public models such as ChatGPT and Google Bard at the forefront of conversations and news. However, AI’s influence on business and the banking sector has been steadily growing over the years – impacting front, middle, and back-office operations. The global market of AI in banking has grown from $6.82 billion in 2022 to $9.00 billion in 2023 – a number we can only expect to grow in 2024 as technology continues to advance.
While increased revenue, streamlined operations, and substantial cost savings are enticing, AI also opens the door to serious security issues if controls are absent. How can community banks use technology to their full advantage, while also minimizing risk?
What is AI?
Understanding AI and machine learning as concepts is crucial for all institutions planning to implement it or use it in any capacity. The National Institute of Standards and Technology (NIST) defines AI as; “The capability of a device to perform functions that are normally associated with human intelligence such as reasoning, learning, and self-improvement.”
Machine learning is the use of artificial neural networks created through massive programs that allow a computer to “learn” new concepts based on the information it is given. AI is essentially the next advancement of machine learning and applies machine learning in a manner that attempts to simulate human reasoning, rational thinking, and problem-solving abilities. The data put out through the AI machine learning model is called “training data,” and data fed to the model by users is called “training sets.” In public AI models, such as ChatGPT, all data that is input by all users is used to help advance the model and “teach” it how to perform more accurately. This is a simple, but extremely important concept to remember. Any information fed into the tool will be stored indefinitely and could be used as part of a response from the AI in future use. As you can imagine, this can pose significant data security threats. With that in mind, organizations also have the option to build their own private model, or to pay for one as a hosted service, which would allow for sensitive information to be processed within the private instance.
How are banks already using AI? What are the future opportunities?
A survey conducted by the University of Cambridge and the World Economic Forum on Global AI in Financial Services found that 85% of respondents are already using some form of AI. Among firms using AI, risk management was the most common application for AI. Firms are most frequently employing AI-enabled analytics, fraud detection and surveillance, and customer communication channels. Considering the advances in AI learning models, these use cases are only going to expand to other business areas.
Addressing the risks associated with AI
Given the way AI is being leveraged, it is understandable that data protection and sharing is a potential risk factor. The Consumer Financial Protection Bureau released an issue this summer on the use of chatbots and the potential risks, including noncompliance with federal consumer financial protection laws, diminished customer service and trust, and harm to consumers.
In addition to complying with evolving regulatory requirements, firms can enhance their internal policies and procedures to help mitigate risk exposure when using AI technologies. Here are a few tips to consider:
- Carefully consider what AI technologies you use or allow access to within your institution. Many large banks have restricted staff use of third-party software, such as Chat GPT, due to potential security issues. Work with your IT and cybersecurity team to understand what data is being exposed to the software, and what protections for data are in place. Consider how you would like to leverage AI, whether for fraud detection, code development, or automating workflows, and conduct a cost benefit analysis to determine if the solution meets your institution’s needs.
- Make staff training a priority. Staff should be trained in what data can be entered into software, and supporting documentation should be readily available for reference. Ensure that all employees with access to the tool(s) understand applicable data protection regulations. AI gathers, processes, and stores substantial amounts of data, which raises concerns about compliance regulations such as GLBA, PCI DSS and CCPA. Mitigating this concern will require ensuring proper data loss prevention (DLP) tools are in place, along with antivirus and network monitoring capabilities.
- Think about where data is stored and what controls are needed. If your bank opts to go with an internal, self-built AI, you should strongly consider air gapping the model and keeping it on its own private network so only those in your organization have access to the data. If you opt to use a hosted version, make sure to compare options and ensure the third party’s cybersecurity posture aligns with your institution’s needs and values. Another major concern is corruption of the AI through poisoning. This means that a bad actor (someone taking a malicious action) gave the AI bad data to manipulate its processing. Proper security controls that log and monitor data input into the system should be in place to reduce risk. Everyone using the AI application should have unique accounts, and all actions should be logged to ensure there is an accurate audit trail.
- Plan your implementation. When developing and implementing internal AI software, remember to follow secure software development procedures. If you are using an application, take measures to ensure it is properly configured and provisioned. At the very least, a risk assessment and business impact analysis should be conducted. Furthermore, incorporating AI into your business continuity plan is strongly recommended. An acceptable use policy (AUP) should also be documented and examined by legal experts, to ensure all users know and understand the organizational polices surrounding the technology. The AUP will enable your institution to document the appropriate use cases for employees in a secure manner. It also will disclose the disciplinary actions that the bank will take if it is violated. Continuous monitoring and maintenance will be required throughout the life span of the AI tool.
Moving forward: seizing opportunities AND staying safe
To adequately prepare, it’s a lot of work and a big investment. But that is nothing compared to the cost of not taking it seriously. The McKinsey Institute estimates that generative AI could add between $200 and $340 billion in value across banking – wholesale and retail. Missing out could have devastating impacts. AI can’t and shouldn’t be ignored. There are benefits that can help improve an institution’s operations, managing employees, and attracting and engaging customers.
Careful planning and understanding the concerns associated with AI applications will continue to be critical to help banks and financial institutions reap the benefits of the technologies while minimizing risk.
Disclaimer of Liability: This publication is intended to provide general information to our clients and friends. It does not constitute accounting, tax, investment, or legal advice; nor is it intended to convey a thorough treatment of the subject matter.